c-suite blog: How to Reduce the Cost to Originate Your Mortgage Loans with Data Analytics
- Galen Okazaki
- Dec 15, 2021
- 4 min read
Updated: Dec 16, 2021
The key to an efficient process is the elimination of as much variation as possible. However, for those of us in the mortgage industry, we know that variation is inherent in every loan we originate. Beginning with the borrower, each loan has its own, unique, set of characteristics. Different properties, different loan programs, different loan officers, the variables for each loan are endless. So how do we as an industry address the reduction of variation within our loan origination process in a cost-effective manner? Quite simply, the answer lies within the data we collect.
For every mortgage loan your company originates, dozens, possibly hundreds, of data points are created and stored. Hidden amongst the terabytes of data that you are collecting are patterns. If you know how to look for them, these patterns can speak volumes about how efficient (or not) your company’s loan origination processes are.
Curious? Let’s look at an example. On average, it takes 30 days for a loan to make it through your pipeline, application to funding. But this past month, over 10% of your fundings were outliers that took over 40 days to fund. We’ll use correlation analysis to determine what characteristics these outliers had in common. We quickly find that the vast majority of them came from one branch and they occurred during the processing stage. We see that these loans had different loan officer/processor combinations, so we know that the delays were not tied to an issue between a specific loan officer and processor. We also find that these loans were spread across different loan programs, so we can eliminate the cause being a training issue with a specific loan program. The number of conditions on these loans fell within the company average, so we know that excessive conditions were not the cause. What we do find, however, is that the same appraiser was used for all of these loans. With the likely root cause having already been identified for us, we now just need to investigate and resolve the issue with the appraiser in question.
While this is a simplistic example, there are two important takeaways from it. First, it introduces the concept of putting into place an organized framework of computer algorithms that can systematically monitor your pipeline for outliers and identify for you what the potential causes might be. In this case, an algorithm did the majority of the root cause analysis without human intervention. The second takeaway is a bit more subtle. Be open-minded to the possibility that these algorithms can lead you to answers that you might not have intuitively looked for in the first place.
So, what should be your first step be if you would like to put something like this into place? First and foremost, you need to identify what your outliers are. What outliers are costing you money? If your company already has a set of Key Performance Indicators (KPI) for the loan origination process, that would be a great place to start. Building on the example above, if your KPI for funding application is 30 days, we can make our analysis more precise by establishing KPIs for each step of the process. Time in processing, time in underwriting, time in closing, etc. With your KPIs in place, you can now start with the next step of developing your team’s analytic skill set.
Building your team’s analytic skill set is an important step. Oftentimes, a company will go out and spend significant capital on building server farms and hiring data scientists (who may not understand the nuances of your business), without truly understanding what it is they would like to analyze. The analysis that I have outlined above, can start with something as simple as a data dump from your loan origination system into Excel. A sharp analyst can perform correlation analysis in Excel or other ubiquitous analytics tools like Tableau or Power BI. By conducting their analytics in this somewhat manual fashion, your team will develop their knowledge of what data is available, where to get it from and how to work with it. You will also get the added benefit of the results of their analysis as they learn.
Once your team gets to the point where repeatable, value add analytics have been developed, you can now look at systematizing it in the form of computer algorithms. The work that your analyst(s) have done will serve as a blueprint for your algorithms. Without getting into an esoteric discussion about how best to do this, just know that the analytics your team has developed can be codified at the server level. The eventual goal should be to have as much of your originations process analytics systematized as possible. It will provide your company the ability to constantly surveil and quickly identify and address potential problem areas within your operational processes.
While the technology barometer, Gartner, has identified the use of analytics to improve a company’s operational processes as one of the fastest-growing trends, we in the mortgage industry have been very slow to adopt it. I believe that the potential benefits are huge and not as costly or complex to attain as you might think. Hopefully, this article can provide you with a place to start. Please feel free to contact me directly at galen@vectordecisionsupport.com with any questions or comments you might have. I look forward to hearing from you.
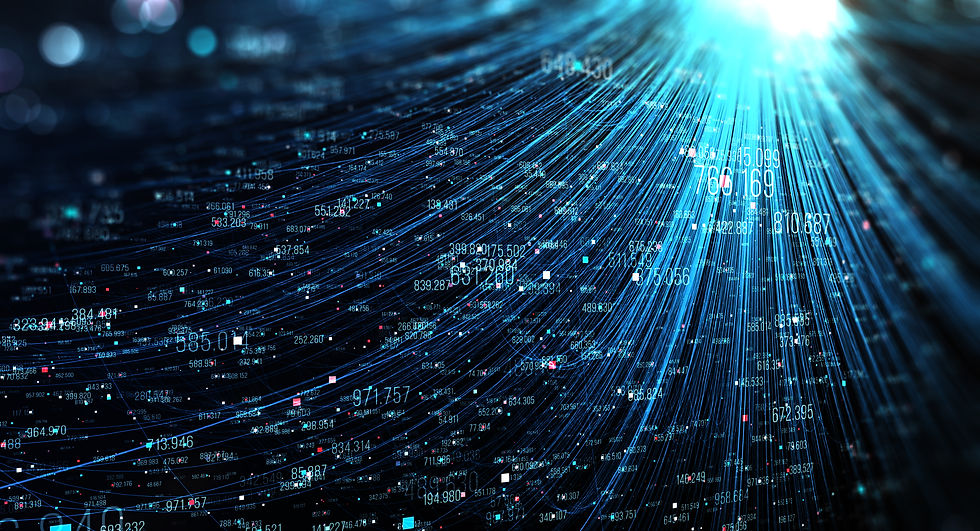
Comentários